Hands-on#
Once we know a bit about what computational notebooks are and why we should care about them, let’s jump to using them! This section introduces you to using Python for manipulating tabular data. Please read through it carefully and pay attention to how ideas about manipulating data are translated into Python code that “does stuff”. For this part, you can read directly from the course website, although it is recommended you follow the section interactively by running code on your own.
Once you have read through and have a bit of a sense of how things work, jump on the Do-It-Yourself section, which will provide you with a challenge to complete it on your own, and will allow you to put what you have already learnt to good use. Happy hacking!
Data munging#
Real world datasets are messy. There is no way around it: datasets have “holes” (missing data), the amount of formats in which data can be stored is endless, and the best structure to share data is not always the optimum to analyze them, hence the need to munge them. As has been correctly pointed out in many outlets (e.g.), much of the time spent in what is called (Geo-)Data Science is related not only to sophisticated modeling and insight, but has to do with much more basic and less exotic tasks such as obtaining data, processing, turning them into a shape that makes analysis possible, and exploring it to get to know their basic properties.
For how labor intensive and relevant this aspect is, there is surprisingly very little published on patterns, techniques, and best practices for quick and efficient data cleaning, manipulation, and transformation. In this session, you will use a few real world datasets and learn how to process them into Python so they can be transformed and manipulated, if necessary, and analyzed. For this, we will introduce some of the bread and butter of data analysis and scientific computing in Python. These are fundamental tools that are constantly used in almost any task relating to data analysis.
This notebook covers the basic and the content that is expected to be learnt by every student. We use a prepared dataset that saves us much of the more intricate processing that goes beyond the introductory level the session is aimed at. As a companion to this introduction, there is an additional notebook (see link on the website page for Lab 01) that covers how the dataset used here was prepared from raw data downloaded from the internet, and includes some additional exercises you can do if you want dig deeper into the content of this lab.
In this notebook, we discuss several patterns to clean and structure data properly, including tidying, subsetting, and aggregating; and we finish with some basic visualization. An additional extension presents more advanced tricks to manipulate tabular data.
Before we get our hands data-dirty, let us import all the additional libraries we will need, so we can get that out of the way and focus on the task at hand:
# This ensures visualizations are plotted inside the notebook
%matplotlib inline
import os # This provides several system utilities
import pandas as pd # This is the workhorse of data munging in Python
import seaborn as sns # This allows us to efficiently and beautifully plot
Dataset#
We will be exploring some demographic characteristics in Liverpool. To do that, we will use a dataset that contains population counts, split by ethnic origin. These counts are aggregated at the Lower Layer Super Output Area (LSOA from now on). LSOAs are an official Census geography defined by the Office of National Statistics. You can think of them, more or less, as neighbourhoods. Many data products (Census, deprivation indices, etc.) use LSOAs as one of their main geographies.
To make things easier, we will read data from a file posted online so, for now, you do not need to download any dataset:
# Read table
db = pd.read_csv("https://darribas.org/gds_course/content/data/liv_pop.csv",
index_col='GeographyCode')
Let us stop for a minute to learn how we have read the file. Here are the main aspects to keep in mind:
We are using the method
read_csv
from thepandas
library, which we have imported with the aliaspd
.In this form, all that is required is to pass the path to the file we want to read, which in this case is a web address.
The argument
index_col
is not strictly necessary but allows us to choose one of the columns as the index of the table. More on indices below.We are using
read_csv
because the file we want to read is in thecsv
format. However,pandas
allows for many more formats to be read and write. A full list of formats supported may be found here.To ensure we can access the data we have read, we store it in an object that we call
db
. We will see more on what we can do with it below but, for now, just keep in mind that allows us to save the result ofread_csv
.
Alternative
Instead of reading the file directly off the web, it is possible to download it manually, store it on your computer, and read it locally. To do that, you can follow these steps:
Download the file by right-clicking on this link and saving the file
Place the file on the same folder as the notebook where you intend to read it
Replace the code in the cell above by:
db = pd.read_csv("liv_pop.csv", index_col="GeographyCode")
Data, sliced and diced#
Now we are ready to start playing and interrogating the dataset! What we have at our fingertips is a table that summarizes, for each of the LSOAs in Liverpool, how many people live in each, by the region of the world where they were born. We call these tables DataFrame
objects, and they have a lot of functionality built-in to explore and manipulate the data they contain. Let’s explore a few of those cool tricks!
Structure#
The first aspect worth spending a bit of time is the structure of a DataFrame
. We can print it by simply typing its name:
db
Europe | Africa | Middle East and Asia | The Americas and the Caribbean | Antarctica and Oceania | |
---|---|---|---|---|---|
GeographyCode | |||||
E01006512 | 910 | 106 | 840 | 24 | 0 |
E01006513 | 2225 | 61 | 595 | 53 | 7 |
E01006514 | 1786 | 63 | 193 | 61 | 5 |
E01006515 | 974 | 29 | 185 | 18 | 2 |
E01006518 | 1531 | 69 | 73 | 19 | 4 |
... | ... | ... | ... | ... | ... |
E01033764 | 2106 | 32 | 49 | 15 | 0 |
E01033765 | 1277 | 21 | 33 | 17 | 3 |
E01033766 | 1028 | 12 | 20 | 8 | 7 |
E01033767 | 1003 | 29 | 29 | 5 | 1 |
E01033768 | 1016 | 69 | 111 | 21 | 6 |
298 rows × 5 columns
Note the printing is cut to keep a nice and compact view, but enough to see its structure. Since they represent a table of data, DataFrame
objects have two dimensions: rows and columns. Each of these is automatically assigned a name in what we will call its index. When printing, the index of each dimension is rendered in bold, as opposed to the standard rendering for the content. In the example above, we can see how the column index is automatically picked up from the .csv
file’s column names. For rows, we have specified when reading the file we wanted the column GeographyCode
, so that is used. If we hadn’t specified any, pandas
will automatically generate a sequence starting in 0
and going all the way to the number of rows minus one. This is the standard structure of a DataFrame
object, so we will come to it over and over. Importantly, even when we move to spatial data, our datasets will have a similar structure.
One final feature that is worth mentioning about these tables is that they can hold columns with different types of data. In our example, this is not used as we have counts (or int
, for integer, types) for each column. But it is useful to keep in mind we can combine this with columns that hold other type of data such as categories, text (str
, for string), dates or, as we will see later in the course, geographic features.
Inspect#
Inspecting what it looks like. We can check the top (bottom) X lines of the table by passing X to the method head
(tail
). For example, for the top/bottom five lines:
db.head()
Europe | Africa | Middle East and Asia | The Americas and the Caribbean | Antarctica and Oceania | |
---|---|---|---|---|---|
GeographyCode | |||||
E01006512 | 910 | 106 | 840 | 24 | 0 |
E01006513 | 2225 | 61 | 595 | 53 | 7 |
E01006514 | 1786 | 63 | 193 | 61 | 5 |
E01006515 | 974 | 29 | 185 | 18 | 2 |
E01006518 | 1531 | 69 | 73 | 19 | 4 |
db.tail()
Europe | Africa | Middle East and Asia | The Americas and the Caribbean | Antarctica and Oceania | |
---|---|---|---|---|---|
GeographyCode | |||||
E01033764 | 2106 | 32 | 49 | 15 | 0 |
E01033765 | 1277 | 21 | 33 | 17 | 3 |
E01033766 | 1028 | 12 | 20 | 8 | 7 |
E01033767 | 1003 | 29 | 29 | 5 | 1 |
E01033768 | 1016 | 69 | 111 | 21 | 6 |
Or getting an overview of the table:
db.info()
<class 'pandas.core.frame.DataFrame'>
Index: 298 entries, E01006512 to E01033768
Data columns (total 5 columns):
# Column Non-Null Count Dtype
--- ------ -------------- -----
0 Europe 298 non-null int64
1 Africa 298 non-null int64
2 Middle East and Asia 298 non-null int64
3 The Americas and the Caribbean 298 non-null int64
4 Antarctica and Oceania 298 non-null int64
dtypes: int64(5)
memory usage: 14.0+ KB
Summarise#
Or of the values of the table:
db.describe()
Europe | Africa | Middle East and Asia | The Americas and the Caribbean | Antarctica and Oceania | |
---|---|---|---|---|---|
count | 298.00000 | 298.000000 | 298.000000 | 298.000000 | 298.000000 |
mean | 1462.38255 | 29.818792 | 62.909396 | 8.087248 | 1.949664 |
std | 248.67329 | 51.606065 | 102.519614 | 9.397638 | 2.168216 |
min | 731.00000 | 0.000000 | 1.000000 | 0.000000 | 0.000000 |
25% | 1331.25000 | 7.000000 | 16.000000 | 2.000000 | 0.000000 |
50% | 1446.00000 | 14.000000 | 33.500000 | 5.000000 | 1.000000 |
75% | 1579.75000 | 30.000000 | 62.750000 | 10.000000 | 3.000000 |
max | 2551.00000 | 484.000000 | 840.000000 | 61.000000 | 11.000000 |
Note how the output is also a DataFrame
object, so you can do with it the same things you would with the original table (e.g. writing it to a file).
In this case, the summary might be better presented if the table is “transposed”:
db.describe().T
count | mean | std | min | 25% | 50% | 75% | max | |
---|---|---|---|---|---|---|---|---|
Europe | 298.0 | 1462.382550 | 248.673290 | 731.0 | 1331.25 | 1446.0 | 1579.75 | 2551.0 |
Africa | 298.0 | 29.818792 | 51.606065 | 0.0 | 7.00 | 14.0 | 30.00 | 484.0 |
Middle East and Asia | 298.0 | 62.909396 | 102.519614 | 1.0 | 16.00 | 33.5 | 62.75 | 840.0 |
The Americas and the Caribbean | 298.0 | 8.087248 | 9.397638 | 0.0 | 2.00 | 5.0 | 10.00 | 61.0 |
Antarctica and Oceania | 298.0 | 1.949664 | 2.168216 | 0.0 | 0.00 | 1.0 | 3.00 | 11.0 |
Equally, common descriptive statistics are also available:
# Obtain minimum values for each table
db.min()
Europe 731
Africa 0
Middle East and Asia 1
The Americas and the Caribbean 0
Antarctica and Oceania 0
dtype: int64
# Obtain minimum value for the column `Europe`
db['Europe'].min()
731
Note here how we have restricted the calculation of the maximum value to one column only.
Similarly, we can restrict the calculations to a single row:
# Obtain standard deviation for the row `E01006512`,
# which represents a particular LSOA
db.loc['E01006512', :].std()
457.8842648530303
Create new columns#
We can generate new variables by applying operations on existing ones. For example, we can calculate the total population by area. Here is a couple of ways to do it:
# Longer, hardcoded
total = db['Europe'] + db['Africa'] + db['Middle East and Asia'] + \
db['The Americas and the Caribbean'] + db['Antarctica and Oceania']
# Print the top of the variable
total.head()
GeographyCode
E01006512 1880
E01006513 2941
E01006514 2108
E01006515 1208
E01006518 1696
dtype: int64
# One shot
total = db.sum(axis=1)
# Print the top of the variable
total.head()
GeographyCode
E01006512 1880
E01006513 2941
E01006514 2108
E01006515 1208
E01006518 1696
dtype: int64
Note how we are using the command sum
, just like we did with max
or min
before but, in this case, we are not applying it over columns (e.g. the max of each column), but over rows, so we get the total sum of populations by areas.
Once we have created the variable, we can make it part of the table:
db['Total'] = total
db.head()
Europe | Africa | Middle East and Asia | The Americas and the Caribbean | Antarctica and Oceania | Total | |
---|---|---|---|---|---|---|
GeographyCode | ||||||
E01006512 | 910 | 106 | 840 | 24 | 0 | 1880 |
E01006513 | 2225 | 61 | 595 | 53 | 7 | 2941 |
E01006514 | 1786 | 63 | 193 | 61 | 5 | 2108 |
E01006515 | 974 | 29 | 185 | 18 | 2 | 1208 |
E01006518 | 1531 | 69 | 73 | 19 | 4 | 1696 |
A different spin on this is assigning new values: we can generate new variables with scalars, and modify those:
# New variable with all ones
db['ones'] = 1
db.head()
Europe | Africa | Middle East and Asia | The Americas and the Caribbean | Antarctica and Oceania | Total | ones | |
---|---|---|---|---|---|---|---|
GeographyCode | |||||||
E01006512 | 910 | 106 | 840 | 24 | 0 | 1880 | 1 |
E01006513 | 2225 | 61 | 595 | 53 | 7 | 2941 | 1 |
E01006514 | 1786 | 63 | 193 | 61 | 5 | 2108 | 1 |
E01006515 | 974 | 29 | 185 | 18 | 2 | 1208 | 1 |
E01006518 | 1531 | 69 | 73 | 19 | 4 | 1696 | 1 |
And we can modify specific values too:
db.loc['E01006512', 'ones'] = 3
db.head()
Europe | Africa | Middle East and Asia | The Americas and the Caribbean | Antarctica and Oceania | Total | ones | |
---|---|---|---|---|---|---|---|
GeographyCode | |||||||
E01006512 | 910 | 106 | 840 | 24 | 0 | 1880 | 3 |
E01006513 | 2225 | 61 | 595 | 53 | 7 | 2941 | 1 |
E01006514 | 1786 | 63 | 193 | 61 | 5 | 2108 | 1 |
E01006515 | 974 | 29 | 185 | 18 | 2 | 1208 | 1 |
E01006518 | 1531 | 69 | 73 | 19 | 4 | 1696 | 1 |
Delete columns#
Permanently deleting variables is also within reach of one command:
del db['ones']
db.head()
Europe | Africa | Middle East and Asia | The Americas and the Caribbean | Antarctica and Oceania | Total | |
---|---|---|---|---|---|---|
GeographyCode | ||||||
E01006512 | 910 | 106 | 840 | 24 | 0 | 1880 |
E01006513 | 2225 | 61 | 595 | 53 | 7 | 2941 |
E01006514 | 1786 | 63 | 193 | 61 | 5 | 2108 |
E01006515 | 974 | 29 | 185 | 18 | 2 | 1208 |
E01006518 | 1531 | 69 | 73 | 19 | 4 | 1696 |
Index-based queries#
Here we explore how we can subset parts of a DataFrame
if we know exactly which bits we want. For example, if we want to extract the total and European population of the first four areas in the table, we use loc
with lists:
eu_tot_first4 = db.loc[['E01006512', 'E01006513', 'E01006514', 'E01006515'], \
['Total', 'Europe']]
eu_tot_first4
Total | Europe | |
---|---|---|
GeographyCode | ||
E01006512 | 1880 | 910 |
E01006513 | 2941 | 2225 |
E01006514 | 2108 | 1786 |
E01006515 | 1208 | 974 |
Note that we use squared brackets ([]
) to delineate the index of the items we want to subset. In Python, this sequence of items is called a list. Hence we can see how we can create a list with the names (index IDs) along each of the two dimensions of a DataFrame
(rows and columns), and loc
will return a subset of the original table only with the elements queried for.
An alternative to list-based queries is what is called “range-based” queries. These work on the indices of the table but, instead of requiring the ID of each item we want to retrieve, the operate by requiring only two IDs: the first and last element in a range of items. Range queries are expressed with a colon (:
). For example:
range_qry = db.loc["E01006514":"E01006518", "Europe":"Antarctica and Oceania"]
range_qry
Europe | Africa | Middle East and Asia | The Americas and the Caribbean | Antarctica and Oceania | |
---|---|---|---|---|---|
GeographyCode | |||||
E01006514 | 1786 | 63 | 193 | 61 | 5 |
E01006515 | 974 | 29 | 185 | 18 | 2 |
E01006518 | 1531 | 69 | 73 | 19 | 4 |
We see how the range query picks up all the elements in between the two IDs specificed. Note that, for this to work, the first ID in the range needs to be placed before the second one in the table’s index.
Once we know about list and range based queries, we can combine them both! For example, we can specify a range of rows and a list of columns:
range_list_qry = db.loc["E01006514":"E01006518", ["Europe", "Total"]]
range_list_qry
Europe | Total | |
---|---|---|
GeographyCode | ||
E01006514 | 1786 | 2108 |
E01006515 | 974 | 1208 |
E01006518 | 1531 | 1696 |
Condition-based queries#
However, sometimes, we do not know exactly which observations we want, but we do know what conditions they need to satisfy (e.g. areas with more than 2,000 inhabitants). For these cases, DataFrames
support selection based on conditions. Let us see a few examples. Suppose we want to select…
… areas with more than 2,500 people in Total:
m5k = db.loc[db['Total'] > 2500, :]
m5k
Europe | Africa | Middle East and Asia | The Americas and the Caribbean | Antarctica and Oceania | Total | |
---|---|---|---|---|---|---|
GeographyCode | ||||||
E01006513 | 2225 | 61 | 595 | 53 | 7 | 2941 |
E01006747 | 2551 | 163 | 812 | 24 | 2 | 3552 |
E01006751 | 1843 | 139 | 568 | 21 | 1 | 2572 |
… areas where there are no more than 750 Europeans:
nm5ke = db.loc[db['Europe'] < 750, :]
nm5ke
Europe | Africa | Middle East and Asia | The Americas and the Caribbean | Antarctica and Oceania | Total | |
---|---|---|---|---|---|---|
GeographyCode | ||||||
E01033757 | 731 | 39 | 223 | 29 | 3 | 1025 |
… areas with exactly ten person from Antarctica and Oceania:
oneOA = db.loc[db['Antarctica and Oceania'] == 10, :]
oneOA
Europe | Africa | Middle East and Asia | The Americas and the Caribbean | Antarctica and Oceania | Total | |
---|---|---|---|---|---|---|
GeographyCode | ||||||
E01006679 | 1353 | 484 | 354 | 31 | 10 | 2232 |
Pro-tip: these queries can grow in sophistication with almost no limits. For example, here is a case where we want to find out the areas where European population is less than half the population:
eu_lth = db.loc[(db['Europe'] * 100. / db['Total']) < 50, :]
eu_lth
Europe | Africa | Middle East and Asia | The Americas and the Caribbean | Antarctica and Oceania | Total | |
---|---|---|---|---|---|---|
GeographyCode | ||||||
E01006512 | 910 | 106 | 840 | 24 | 0 | 1880 |
All the condition-based queries above are expressed using the loc
operator. This is a powerful way and, since it shares syntax with index-based queries, it is also easier to remember. However, sometimes querying using loc
involves a lot of quotation marks, parenthesis, etc. A more streamlined approach for condition-based queries of rows is provided by the query
engine. Using this approach, we express everything in our query on a single string, or piece of text, and that is evaluated in the table at once. For example, we can run the same operation as in the first query above with the following syntax:
m5k_query = db.query("Total > 2500")
If we want to combine operations, this is also possible:
m5k_query2 = db.query("(Total > 2500) & (Total < 10000)")
Note that, in these cases, using query
results in code that is much more streamlined and easier to read. However, query
is not perfect and, particularly for more sophisticated queries, it does not afford the same degree of flexibility. For example, the last query we had using loc
would not be possible using query
.
Combining queries#
Now all of these queries can be combined with each other, for further flexibility. For example, imagine we want areas with more than 25 people from the Americas and Caribbean, but less than 1,500 in total:
ac25_l500 = db.loc[(db['The Americas and the Caribbean'] > 25) & \
(db['Total'] < 1500), :]
ac25_l500
Europe | Africa | Middle East and Asia | The Americas and the Caribbean | Antarctica and Oceania | Total | |
---|---|---|---|---|---|---|
GeographyCode | ||||||
E01033750 | 1235 | 53 | 129 | 26 | 5 | 1448 |
E01033752 | 1024 | 19 | 114 | 33 | 6 | 1196 |
E01033754 | 1262 | 37 | 112 | 32 | 9 | 1452 |
E01033756 | 886 | 31 | 221 | 42 | 5 | 1185 |
E01033757 | 731 | 39 | 223 | 29 | 3 | 1025 |
E01033761 | 1138 | 52 | 138 | 33 | 11 | 1372 |
Sorting#
Among the many operations DataFrame
objects support, one of the most useful ones is to sort a table based on a given column. For example, imagine we want to sort the table by total population:
db_pop_sorted = db.sort_values('Total', ascending=False)
db_pop_sorted.head()
Europe | Africa | Middle East and Asia | The Americas and the Caribbean | Antarctica and Oceania | Total | |
---|---|---|---|---|---|---|
GeographyCode | ||||||
E01006747 | 2551 | 163 | 812 | 24 | 2 | 3552 |
E01006513 | 2225 | 61 | 595 | 53 | 7 | 2941 |
E01006751 | 1843 | 139 | 568 | 21 | 1 | 2572 |
E01006524 | 2235 | 36 | 125 | 24 | 11 | 2431 |
E01006787 | 2187 | 53 | 75 | 13 | 2 | 2330 |
If you inspect the help of db.sort_values
, you will find that you can pass more than one column to sort the table by. This allows you to do so-called hiearchical sorting: sort first based on one column, if equal then based on another column, etc.
Visual exploration#
The next step to continue exploring a dataset is to get a feel for what it looks like, visually. We have already learnt how to unconver and inspect specific parts of the data, to check for particular cases we might be intersted in. Now we will see how to plot the data to get a sense of the overall distribution of values. For that, we will be using the Python library seaborn
.
Histograms.
One of the most common graphical devices to display the distribution of values in a variable is a histogram. Values are assigned into groups of equal intervals, and the groups are plotted as bars rising as high as the number of values into the group.
A histogram is easily created with the following command. In this case, let us have a look at the shape of the overall population:
_ = sns.distplot(db['Total'], kde=False)
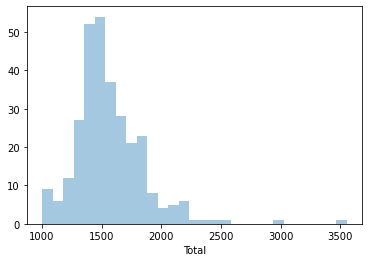
Note we are using sns
instead of pd
, as the function belongs to seaborn
instead of pandas
.
We can quickly see most of the areas contain somewhere between 1,200 and 1,700 people, approx. However, there are a few areas that have many more, even up to 3,500 people.
An additional feature to visualize the density of values is called rug
, and adds a little tick for each value on the horizontal axis:
_ = sns.distplot(db['Total'], kde=False, rug=True)
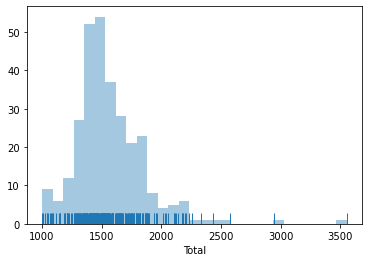
Kernel Density Plots
Histograms are useful, but they are artificial in the sense that a continuous variable is made discrete by turning the values into discrete groups. An alternative is kernel density estimation (KDE), which produces an empirical density function:
_ = sns.kdeplot(db['Total'], shade=True)
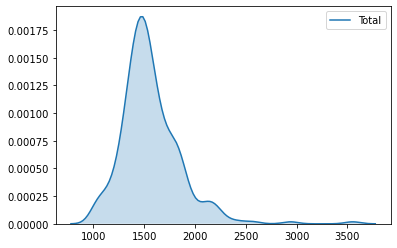
Line and bar plots
Another very common way of visually displaying a variable is with a line or a bar chart. For example, if we want to generate a line plot of the (sorted) total population by area:
_ = db['Total'].sort_values(ascending=False).plot()
/opt/conda/lib/python3.7/site-packages/pandas/plotting/_matplotlib/core.py:1235: UserWarning: FixedFormatter should only be used together with FixedLocator
ax.set_xticklabels(xticklabels)
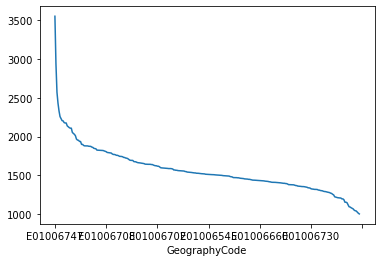
For a bar plot all we need to do is to change from plot
to plot.bar
. Since there are many neighbourhoods, let us plot only the ten largest ones (which we can retrieve with head
):
_ = db['Total'].sort_values(ascending=False)\
.head(10)\
.plot.bar()
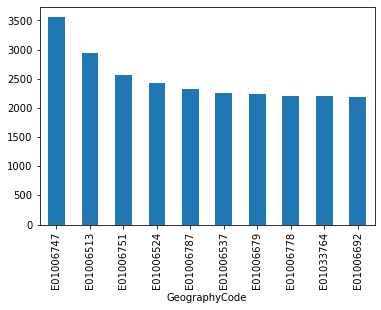
We can turn the plot around by displaying the bars horizontally (see how it’s just changing bar
for barh
). Let’s display now the top 50 areas and, to make it more readable, let us expand the plot’s height:
_ = db['Total'].sort_values()\
.head(50)\
.plot.barh(figsize=(6, 20))
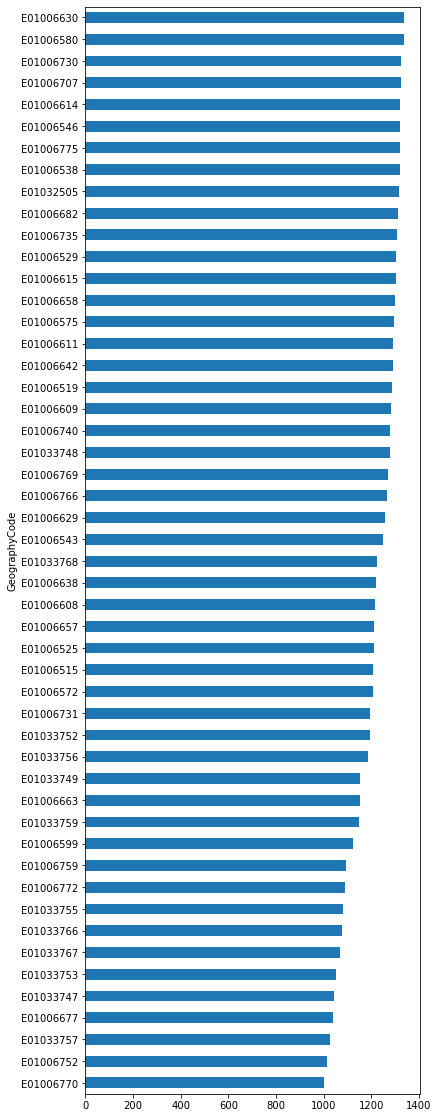
Un/tidy data#
Warning
This section is a bit more advanced and hence considered optional. Fell free to skip it, move to the next, and return later when you feel more confident.
Happy families are all alike; every unhappy family is unhappy in its own way.
Leo Tolstoy.
Once you can read your data in, explore specific cases, and have a first visual approach to the entire set, the next step can be preparing it for more sophisticated analysis. Maybe you are thinking of modeling it through regression, or on creating subgroups in the dataset with particular characteristics, or maybe you simply need to present summary measures that relate to a slightly different arrangement of the data than you have been presented with.
For all these cases, you first need what statistician, and general R wizard, Hadley Wickham calls “tidy data”. The general idea to “tidy” your data is to convert them from whatever structure they were handed in to you into one that allows convenient and standardized manipulation, and that supports directly inputting the data into what he calls “tidy” analysis tools. But, at a more practical level, what is exactly “tidy data”? In Wickham’s own words:
Tidy data is a standard way of mapping the meaning of a dataset to its structure. A dataset is messy or tidy depending on how rows, columns and tables are matched up with observations, variables and types.
He then goes on to list the three fundamental characteristics of “tidy data”:
Each variable forms a column.
Each observation forms a row.
Each type of observational unit forms a table.
If you are further interested in the concept of “tidy data”, I recommend you check out the original paper (open access) and the public repository associated with it.
Let us bring in the concept of “tidy data” to our own Liverpool dataset. First, remember its structure:
db.head()
Europe | Africa | Middle East and Asia | The Americas and the Caribbean | Antarctica and Oceania | Total | |
---|---|---|---|---|---|---|
GeographyCode | ||||||
E01006512 | 910 | 106 | 840 | 24 | 0 | 1880 |
E01006513 | 2225 | 61 | 595 | 53 | 7 | 2941 |
E01006514 | 1786 | 63 | 193 | 61 | 5 | 2108 |
E01006515 | 974 | 29 | 185 | 18 | 2 | 1208 |
E01006518 | 1531 | 69 | 73 | 19 | 4 | 1696 |
Thinking through tidy lenses, this is not a tidy dataset. It is not so for each of the three conditions:
Starting by the last one (each type of observational unit forms a table), this dataset actually contains not one but two observational units: the different areas of Liverpool, captured by
GeographyCode
; and subgroups of an area. To tidy up this aspect, we can create two different tables:
# Assign column `Total` into its own as a single-column table
db_totals = db[['Total']]
db_totals.head()
Total | |
---|---|
GeographyCode | |
E01006512 | 1880 |
E01006513 | 2941 |
E01006514 | 2108 |
E01006515 | 1208 |
E01006518 | 1696 |
# Create a table `db_subgroups` that contains every column in `db` without `Total`
db_subgroups = db.drop('Total', axis=1)
db_subgroups.head()
Europe | Africa | Middle East and Asia | The Americas and the Caribbean | Antarctica and Oceania | |
---|---|---|---|---|---|
GeographyCode | |||||
E01006512 | 910 | 106 | 840 | 24 | 0 |
E01006513 | 2225 | 61 | 595 | 53 | 7 |
E01006514 | 1786 | 63 | 193 | 61 | 5 |
E01006515 | 974 | 29 | 185 | 18 | 2 |
E01006518 | 1531 | 69 | 73 | 19 | 4 |
Note we use drop
to exclude “Total”, but we could also use a list with the names of all the columns to keep. Additionally, notice how, in this case, the use of drop
(which leaves db
untouched) is preferred to that of del
(which permanently removes the column from db
).
At this point, the table db_totals
is tidy: every row is an observation, every table is a variable, and there is only one observational unit in the table.
The other table (db_subgroups
), however, is not entirely tidied up yet: there is only one observational unit in the table, true; but every row is not an observation, and there are variable values as the names of columns (in other words, every column is not a variable). To obtain a fully tidy version of the table, we need to re-arrange it in a way that every row is a population subgroup in an area, and there are three variables: GeographyCode
, population subgroup, and population count (or frequency).
Because this is actually a fairly common pattern, there is a direct way to solve it in pandas
:
tidy_subgroups = db_subgroups.stack()
tidy_subgroups.head()
GeographyCode
E01006512 Europe 910
Africa 106
Middle East and Asia 840
The Americas and the Caribbean 24
Antarctica and Oceania 0
dtype: int64
The method stack
, well, “stacks” the different columns into rows. This fixes our “tidiness” problems but the type of object that is returning is not a DataFrame
:
type(tidy_subgroups)
pandas.core.series.Series
It is a Series
, which really is like a DataFrame
, but with only one column. The additional information (GeographyCode
and population group) are stored in what is called an multi-index. We will skip these for now, so we would really just want to get a DataFrame
as we know it out of the Series
. This is also one line of code away:
# Unfold the multi-index into different, new columns
tidy_subgroupsDF = tidy_subgroups.reset_index()
tidy_subgroupsDF.head()
GeographyCode | level_1 | 0 | |
---|---|---|---|
0 | E01006512 | Europe | 910 |
1 | E01006512 | Africa | 106 |
2 | E01006512 | Middle East and Asia | 840 |
3 | E01006512 | The Americas and the Caribbean | 24 |
4 | E01006512 | Antarctica and Oceania | 0 |
To which we can apply to renaming to make it look better:
tidy_subgroupsDF = tidy_subgroupsDF.rename(columns={'level_1': 'Subgroup', 0: 'Freq'})
tidy_subgroupsDF.head()
GeographyCode | Subgroup | Freq | |
---|---|---|---|
0 | E01006512 | Europe | 910 |
1 | E01006512 | Africa | 106 |
2 | E01006512 | Middle East and Asia | 840 |
3 | E01006512 | The Americas and the Caribbean | 24 |
4 | E01006512 | Antarctica and Oceania | 0 |
Now our table is fully tidied up!
Grouping, transforming, aggregating#
One of the advantage of tidy datasets is they allow to perform advanced transformations in a more direct way. One of the most common ones is what is called “group-by” operations. Originated in the world of databases, these operations allow you to group observations in a table by one of its labels, index, or category, and apply operations on the data group by group.
For example, given our tidy table with population subgroups, we might want to compute the total sum of population by each group. This task can be split into two different ones:
Group the table in each of the different subgroups.
Compute the sum of
Freq
for each of them.
To do this in pandas
, meet one of its workhorses, and also one of the reasons why the library has become so popular: the groupby
operator.
pop_grouped = tidy_subgroupsDF.groupby('Subgroup')
pop_grouped
<pandas.core.groupby.generic.DataFrameGroupBy object at 0x7f3d01696ad0>
The object pop_grouped
still hasn’t computed anything, it is only a convenient way of specifying the grouping. But this allows us then to perform a multitude of operations on it. For our example, the sum is calculated as follows:
pop_grouped.sum()
Freq | |
---|---|
Subgroup | |
Africa | 8886 |
Antarctica and Oceania | 581 |
Europe | 435790 |
Middle East and Asia | 18747 |
The Americas and the Caribbean | 2410 |
Similarly, you can also obtain a summary of each group:
pop_grouped.describe()
Freq | ||||||||
---|---|---|---|---|---|---|---|---|
count | mean | std | min | 25% | 50% | 75% | max | |
Subgroup | ||||||||
Africa | 298.0 | 29.818792 | 51.606065 | 0.0 | 7.00 | 14.0 | 30.00 | 484.0 |
Antarctica and Oceania | 298.0 | 1.949664 | 2.168216 | 0.0 | 0.00 | 1.0 | 3.00 | 11.0 |
Europe | 298.0 | 1462.382550 | 248.673290 | 731.0 | 1331.25 | 1446.0 | 1579.75 | 2551.0 |
Middle East and Asia | 298.0 | 62.909396 | 102.519614 | 1.0 | 16.00 | 33.5 | 62.75 | 840.0 |
The Americas and the Caribbean | 298.0 | 8.087248 | 9.397638 | 0.0 | 2.00 | 5.0 | 10.00 | 61.0 |
We will not get into it today as it goes beyond the basics we want to conver, but keep in mind that groupby
allows you to not only call generic functions (like sum
or describe
), but also your own functions. This opens the door for virtually any kind of transformation and aggregation possible.
Additional lab materials#
The following provide a good “next step” from some of the concepts and tools covered in the lab and DIY sections of this block:
This NY Times article does a good job at conveying the relevance of data “cleaning” and munging.
A good introduction to data manipulation in Python is Wes McKinney’s “Python for Data Analysis” mckinney2012python.
To explore further some of the visualization capabilities in at your fingertips, the Python library
seaborn
is an excellent choice. Its online tutorial is a fantastic place to start.A good extension is Hadley Wickham’ “Tidy data” paper Wickham:2014:JSSOBK:v59i10, which presents a very popular way of organising tabular data for efficient manipulation.